简单的神经网络:全链接
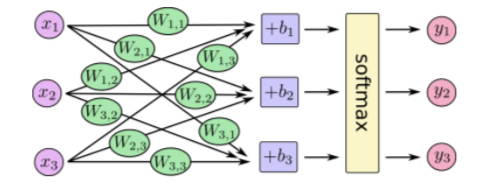
代码解释: def main(_): # Import data # 加载训练数据和测试数据 mnist = input_data.read_data_sets(FLAGS.data_dir, one_hot=True) # Create the model # 定义图像输入变量 x , 作为训练和测试数据的入口,None 表示可输入任意数量的图片,每张图片有 784 个像素,即 784 个 x 变量 x = tf.placeholder(tf.float32, [None, 784]) # 定义变量 x 的参数 w,因为后面会追加偏移量 b,同时输出是 10 个结果集(1到10的可能性概率) W = tf.Variable(tf.zeros([784, 10])) b = tf.Variable(tf.zeros([10])) # 简单的全网络链接,通过矩阵计算输出结果集合 y = tf.matmul(x, W) + b # Define loss and optimizer y_ = tf.placeholder(tf.float32, [None, 10]) # The raw formulation of cross-entropy, # # tf.reduce_mean(-tf.reduce_sum(y_ * tf.log(tf.nn.softmax(y)), # reduction_indices=[1])) # # can be numerically unstable. # # So here we use tf.nn.softmax_cross_entropy_with_logits on the raw # outputs of 'y', and then average across the batch. # 计算模型输出结果和真实结果的误差,采用交叉熵作为损失函数 cross_entropy = tf.reduce_mean( tf.nn.softmax_cross_entropy_with_logits(labels=y_, logits=y)) # 训练步伐,通过求导计算,一点点地逼近损失函数的极值点 train_step = tf.train.GradientDescentOptimizer(0.5).minimize(cross_entropy) # 初始化流程图 sess = tf.InteractiveSession() tf.global_variables_initializer().run() # Train # 开始训练,一共训练 1000 次,每次读取 100 张图片,避免一次性读入太多图片导致内存不足或者计算量过大的问题 for _ in range(1000): batch_xs, batch_ys = mnist.train.next_batch(100) sess.run(train_step, feed_dict={x: batch_xs, y_: batch_ys}) # Test trained model # 测试模型的准确率 correct_prediction = tf.equal(tf.argmax(y, 1), tf.argmax(y_, 1)) accuracy = tf.reduce_mean(tf.cast(correct_prediction, tf.float32)) print(sess.run(accuracy, feed_dict={x: mnist.test.images, y_: mnist.test.labels}))
Leave a Reply